BEYOND THE ORDINARY
Boost Your Business Performance with
Time Series Analysis Solutions
"BlueBash AI excels in harnessing time series analysis to drive business transformation. Our expertise lies in mastering this field, helping organisations leverage data trends and insights for strategic growth."
Let’s Build Your Business Application!
We are a team of top custom software developers, having knowledge-rich experience in developing E-commerce Software and Healthcare software. With years of existence and skills, we have provided IT services to our clients that completely satisfy their requirements.
What We Offer in Time Series Analysis
Trend Analysis
"Trend analysis scrutinizes data patterns within a defined timeframe to predict future trends. Techniques like exponential smoothing, ARIMA, and state-space models are applied. It involves examining historical data to uncover trends, which are then extrapolated into the future."
Market Forecasting
Revenue Projections
Resource Allocation
Seasonality Decomposition
"Seasonality Decomposition dissects time series into trend, seasonal, and residual elements, often utilizing methods like STL (Seasonal-Trend decomposition using LOESS). This process involves isolating seasonal patterns within the data, enabling separate or combined analysis with other factors."
Inventory Planning
Marketing Strategy
Workforce Management
Custom Time Series Models
"Our time series models are custom-crafted to meet your distinct business needs, which may include a fusion of trend and seasonal models or innovative algorithms. We begin with an in-depth consultation to grasp your unique challenge and then engineer a bespoke time series model, rigorously tested and validated."
Tailored Solutions
Optimized ROI
History of Time Series Analysis Machine Learning
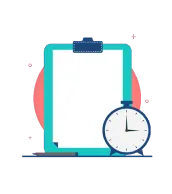
-
Basics & Foundations:
Yule's work on autoregressive models.
-
Seasonal Decompositions:
Introduced to deal with seasonality in economic data.
-
Spectrum Analysis:
Periodogram and spectral density functions are introduced.
-
Exponential Smoothing:
Brown introduces exponential smoothing.
-
ARIMA Models:
Box and Jenkins popularize ARIMA
-
State Space Models:
Kalman Filter used in time series.
-
Machine Learning:
Time series forecasting starts incorporating machine learning techniques like Neural Networks.
-
Big Data:
Time series databases and real-time analytics
-
Real-World Applications:
IoT and sensor data create a plethora of time series data for real-world applications.
Why Bluebash AI for Time Series Analysis?
-
Focused Expertise:
Specialized in data pipelines, offering the most advanced solutions.
-
Quick Deployment:
Rapid integration into your existing systems for immediate benefits.
-
Secure and Scalable:
Designed with data security and scalability in mind.
-
Customer-Centric:
Tailored solutions to meet your unique challenges.
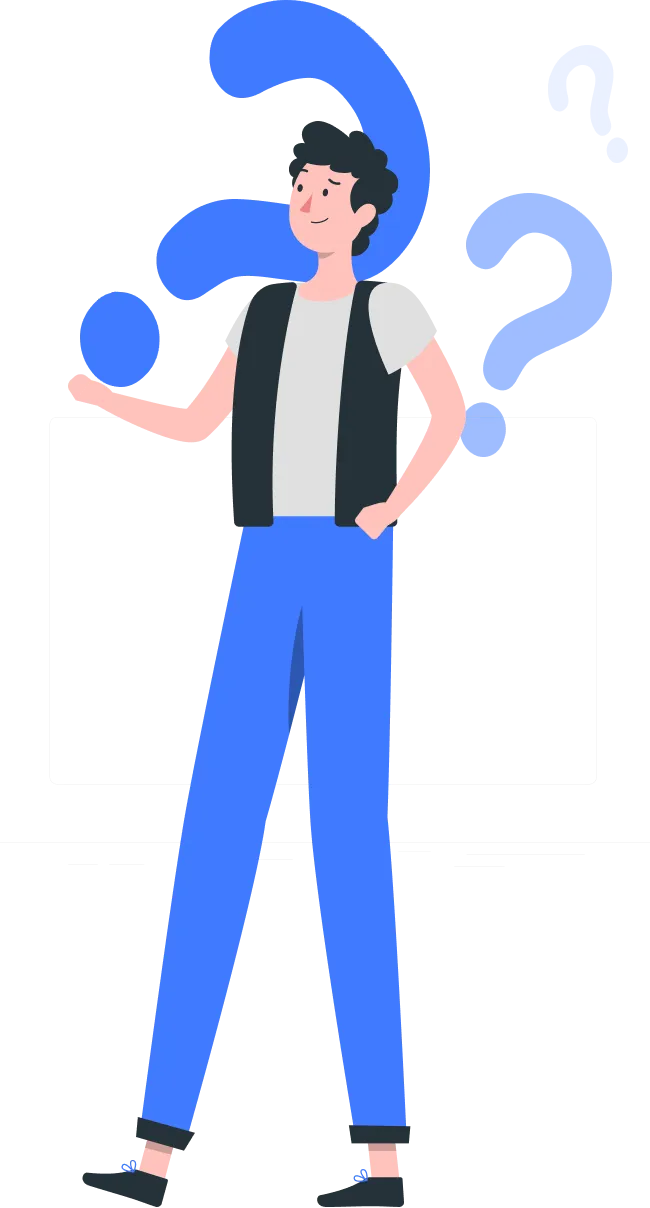
Case Study: Time Series Analysis
Retail: Inventory Planning
BlueBash AI implemented a time series model to forecast demand.
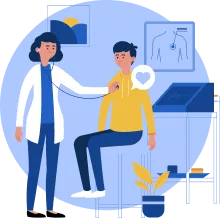
Healthcare: Patient Monitoring
Continuous time series analysis of patient data.
Energy: Consumption Forecasting
BlueBash AI developed a customized time series model.
Frequently Asked Questions
Time series forecasting involves analyzing historical data patterns to predict future values. Our AI-driven solutions analyze past data trends to make accurate predictions for your business needs.
Time series forecasting empowers businesses to make data-driven decisions, anticipate trends, optimize resource allocation, and enhance planning and strategies based on predictive analytics.
Our AI-driven time series analysis software leverages advanced machine learning algorithms, ensuring high accuracy in predicting future values based on historical data patterns.
Yes, our suite of tools includes real-time time series analysis capabilities, allowing for quick and precise predictions even with constantly evolving data.
Absolutely, our time series analysis solutions are adaptable and customizable to suit various industry requirements, ensuring tailored and precise forecasting models.
Our AI-driven software employs cutting-edge algorithms, ensuring higher accuracy and adaptability compared to traditional methods, resulting in more reliable predictions.
Here are the steps to analyze time series: 1. Collect the data and clean it. 2. Prepare visualization with respect to time vs. key feature. 3. Observe the stationarity of the series. 4. Develop charts to understand its nature. 5. Build the model – AR, MA, ARMA, and ARIMA. 6. Extract insights from prediction.